Magic of Variational Autoencoders (VAEs) in Generative AI
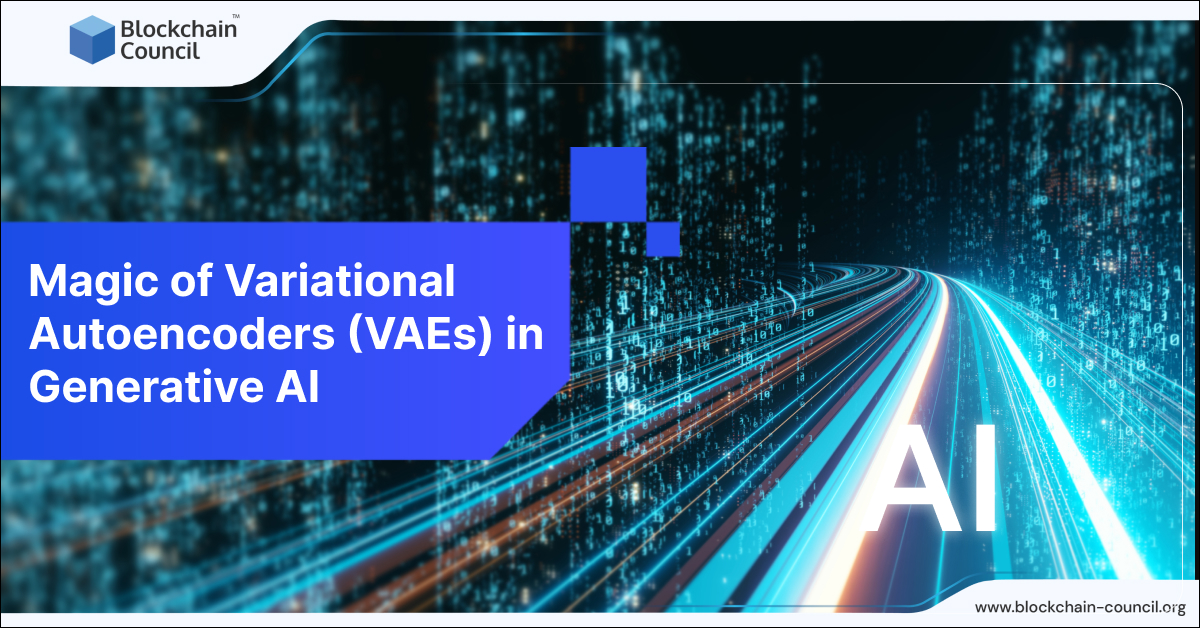
Variational autoencoders (VAEs) have become instrumental in the field of artificial intelligence (AI), offering a unique approach to data processing and synthesis that has garnered significant attention. These sophisticated models, introduced by Diederik P. Kingma and Max Welling in 2013, represent a paradigm shift in the way AI systems understand and generate data.
Understanding Variational Autoencoders (VAEs)
VAEs are a type of artificial neural network designed to learn the underlying structure of datasets and generate new data samples based on this learned structure. Unlike traditional autoencoders, VAEs introduce a probabilistic element, allowing for more flexible and diverse data generation. They achieve this by encoding input data into a lower-dimensional latent space and then decoding it back into the original space, all while adhering to probabilistic principles.
The Evolution of Variational Autoencoders (VAEs)
Since their inception, VAEs have undergone significant evolution, driven by advances in deep learning, probabilistic modelling, and optimization techniques. Researchers and practitioners have continuously refined VAE architectures and training methodologies, enhancing their generative capabilities and applicability across various domains. From image synthesis to natural language processing, VAEs have become indispensable tools in AI research and application.
The Significance of Variational Autoencoders (VAEs)
The significance of VAEs lies in their ability to learn complex data distributions in an unsupervised manner and generate new, coherent samples. This capability is particularly valuable in scenarios where labelled data is scarce or expensive to obtain. VAEs facilitate tasks such as data synthesis, anomaly detection, and representation learning, offering a principled framework for exploring and manipulating high-dimensional data features.
The Mechanism Behind Variational Autoencoders (VAEs)
VAEs operate through a dual process of encoding and decoding. During the encoding phase, input data is mapped to a lower-dimensional latent space, capturing the essential features of the data. In the decoding phase, the latent representation is reconstructed back into the original data space, generating new samples. This process involves a delicate balance between capturing data features accurately and ensuring the diversity of generated samples.
Variational Autoencoders (VAEs) in Practice
In practical applications, VAEs have demonstrated their utility across a wide range of domains. In medical imaging, VAEs have been used for tasks such as anomaly detection, image reconstruction, and disease diagnosis. In natural language processing, VAEs have been applied to text generation, semantic parsing, and language modelling. Additionally, VAEs play a crucial role in cybersecurity, where they aid in anomaly detection and intrusion analysis by learning normal patterns of network traffic and system behaviour.
Assessing the Advantages and Limitations of Variational Autoencoders (VAEs)
While VAEs offer several advantages, including their generative capabilities, unsupervised learning ability, and latent space manipulation, they also have limitations. Challenges such as approximate inference, complex training dynamics, and mode collapse can affect the performance and reliability of VAEs. It is essential to consider these factors when deploying VAEs in real-world applications and to adopt strategies to mitigate potential issues.
Exploring Related Concepts in AI
The landscape of VAEs is intertwined with several related concepts and techniques in AI. Autoencoders, the foundation of VAEs, emphasize the encoding and decoding mechanisms for learning data representations. Generative adversarial networks (GANs), another prominent approach to generative modelling, offer an alternative perspective to VAEs with distinct training dynamics and sample generation techniques. Bayesian inference principles underpin the probabilistic framework of VAEs, influencing their approach to modelling latent variables and uncertainty estimation.
Unlocking Opportunities: The Power of AI Certification for Prompt Engineers and Artificial Intelligence Developers
In the evolving landscape of technology, the importance of AI certification cannot be overstated, particularly for aspiring prompt engineers and AI developers. Artificial intelligence certification programs offer invaluable opportunities for professionals to acquire specialized skills and knowledge essential for thriving in the field of AI development. These AI certification courses provide a structured curriculum covering diverse topics ranging from foundational principles of AI to advanced techniques in prompt engineering and generative AI. By completing an artificial intelligence certification, individuals demonstrate their proficiency in cutting-edge technologies and methodologies, enhancing their credibility and employability in the competitive job market. Furthermore, generative AI certification programs often include hands-on projects and real-world applications, allowing participants to gain practical experience and refine their problem-solving abilities. For aspiring prompt engineers, obtaining a prompt engineering course certification signifies a commitment to continuous learning and professional growth, ensuring they remain at the forefront of technological innovation. Prompt engineer certification serves as a catalyst for career advancement, empowering individuals to unlock new opportunities and make meaningful contributions to the expanding field of artificial intelligence.
Conclusion: The Promise of Variational Autoencoders (VAEs)
VAEs represent a significant advancement in AI, offering a principled approach to unsupervised learning, generative modelling, and latent space exploration. Their impact spans diverse applications, ranging from healthcare and natural language processing to cybersecurity. As VAEs continue to evolve and integrate with cutting-edge AI technologies, their potential to drive innovation and address complex challenges remains substantial, promising a future where AI systems can understand, create, and interpret data with unprecedented sophistication and accuracy.
For those interested in upskilling, Blockchain Council provides prompt engineer course programs, empowering individuals with specialized skills in prompt engineering and AI development. Blockchain Council is a leading platform comprising subject experts and enthusiasts dedicated to advancing research, development, and knowledge dissemination in blockchain technology. With its comprehensive resources and certification programs, Blockchain Council equips professionals with the expertise needed to thrive in the rapidly evolving landscape of artificial intelligence and emerging technologies.